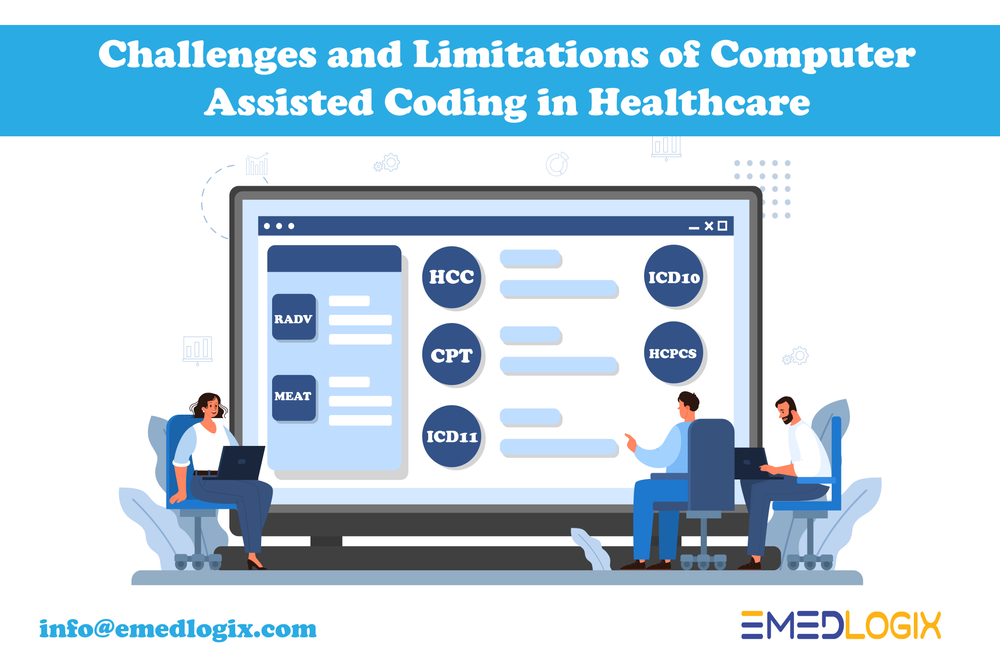
Computer-Assisted Coding (CAC) has emerged as a critical tool in healthcare, aiming to streamline the complex process of medical coding. By automating the coding process, CAC systems promise increased efficiency, accuracy, and reduced administrative burden for healthcare providers. However, like any technological advancement, CAC comes with its own set of challenges and limitations. In this article, we will explore the different types of CAC coding, delve into the challenges associated with its implementation, and discuss the role of Artificial Intelligence (AI) in healthcare, particularly focusing on how generative AI can revolutionize Hierarchical Condition Category (HCC) coding. Additionally, we’ll touch upon the Emedlogix NLP tool and its significance in the healthcare coding landscape.
Types of CAC Coding:
There are two main types of CAC systems:
1. Rule-based systems: These systems operate on predefined coding rules. They are programmed to follow specific guidelines and regulations, making them suitable for structured and well-defined coding scenarios. However, rule-based systems may struggle with adapting to evolving coding practices and may lack the flexibility needed for dynamic healthcare environments.
2. Machine learning systems: These systems leverage machine learning algorithms trained on large datasets to identify patterns and make predictions. Machine learning-based CAC can adapt to changing coding patterns, making them more versatile than rule-based systems. Nevertheless, they require extensive training data and continuous updates to maintain accuracy.
Challenges of CAC Implementation:
Despite the promises of CAC, its implementation faces several challenges:
1. Accuracy and Specificity: CAC systems may struggle with achieving the same level of accuracy and specificity as experienced human coders. Complex cases, unusual scenarios, and ambiguous documentation can pose challenges for automated coding.
2. Integration with Electronic Health Records (EHR): Seamless integration with EHR systems is crucial for the success of CAC. However, achieving interoperability between diverse EHR platforms and CAC solutions can be a significant hurdle.
3. Regulatory Compliance: Adhering to ever-evolving healthcare regulations and coding guidelines is essential. CAC systems must be continuously updated to comply with the latest coding standards, which requires significant resources and effort.
AI in Healthcare and Generative AI in HCC Coding:
Artificial Intelligence is making significant strides in healthcare, and generative AI, in particular, holds promise for transforming HCC coding. Generative AI can understand and generate human-like text, making it a valuable tool for interpreting and coding complex medical documentation.
By utilizing generative AI, HCC coding can benefit from:
1. Enhanced Natural Language Processing: Generative AI can improve the understanding of nuanced medical language, leading to more accurate code assignments for conditions that are often challenging to code manually.
2. Automated Documentation Improvement: Generative AI can assist in generating comprehensive and precise clinical documentation, addressing one of the root causes of coding inaccuracies.
3. Real-time Coding Suggestions: Integrating generative AI into CAC systems can provide real-time coding suggestions, aiding coders in making informed decisions and reducing the risk of errors.
Emedlogix NLP Tool:
Emedlogix’s NLP tool plays a crucial role in overcoming challenges associated with CAC. This NLP tool specializes in extracting meaningful information from unstructured clinical documentation, improving the accuracy and efficiency of coding processes. Its advanced linguistic capabilities make it a valuable asset in the evolving landscape of healthcare coding.
Conclusion:
While CAC systems offer substantial benefits to healthcare organizations, it is essential to recognize and address the challenges they present. Incorporating generative AI, such as Emedlogix’s NLP tool, into the CAC framework can contribute to overcoming these challenges and usher in a new era of efficiency and accuracy in medical coding. As technology continues to advance, the collaboration between AI and healthcare professionals will play a pivotal role in shaping the future of medical coding.
