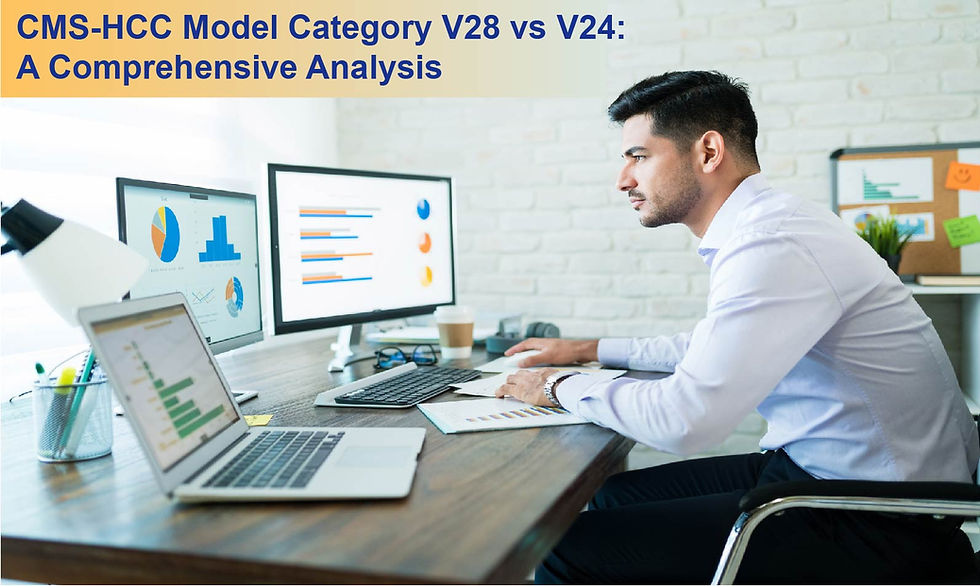
The CMS-HCC (Centers for Medicare & Medicaid Services Hierarchical Condition Categories) model is a risk adjustment model that plays a crucial role in predicting the future healthcare costs of beneficiaries. Over time, this model has undergone several revisions, with V28 and V24 being two of its notable versions. This article delves deep into the differences and nuances between these two versions, drawing insights from both the original CMS document and its subsequent analysis.
1. Introduction to CMS-HCC Model
The CMS-HCC model is designed to predict the future healthcare costs of beneficiaries. It is a risk adjustment model that takes into account various factors, including the beneficiary's health conditions and demographic details. The model has been revised multiple times, with V28 and V24 being two significant versions.
2. Why These Changes?
Risk score differences between the current model and the proposed model occur for several reasons. Specifically, revisions to the models result in changes in the marginal cost attributable to each HCC. These changes can alter the relative factor associated with each HCC and with the relative values among HCCs. Additionally, the new categories and updated HCCs reflect possible changes to physician coding patterns that have developed as a result of the transition to ICD-10. Changes to the condition categories, including additions, deletions, and revisions, are based on each condition category’s ability to predict costs for Medicare Parts A and B benefits.
3. Number of Changes in Codes
The transition from V24 to V28 saw a reclassification of several diagnosis codes. For instance, there were changes in the way certain conditions like diabetes, heart disease, kidney disease, musculoskeletal disease, psychiatric conditions, and vascular disease were categorized between V24 and V28.
Proposed Updates for CY 2024 by CMS
For the calendar year 2024, CMS has put forth a revised Part C CMS-HCC risk adjustment model, specifically tailored for organizations excluding PACE. This proposed model is anticipated to result in a -3.12% impact on MA risk scores. Financially, this translates to an impressive net savings of $11.0 billion for the Medicare Trust fund in the year 2024.
Expansion and Renumbering of HCC Categories
The upcoming model will see an increase in HCC categories, expanding from 86 to a total of 115. Alongside this expansion, there will be a renumbering of these categories.
Understanding V28 HCC Code Nomenclature
The V28 HCC codes have undergone changes in their naming and numbering conventions, reflecting the model's evolution and the need for more precise categorization.
Introduction of Additional HCCs
The new model introduces a broader range of HCCs, ensuring a more comprehensive representation of health conditions.
Modifications in ICD-10-CM Code to HCC Mappings
There have been significant alterations in the mappings from ICD-10-CM codes to HCCs, ensuring that the codes are more aligned with the latest medical understanding and practices.
Adjustments in HCC Coefficient Values
The coefficient values associated with HCCs have been updated, reflecting the changing dynamics of healthcare costs and needs.
Refinement in Diagnosis Code Mappings
The new model has removed 2,294 diagnosis codes that were previously mapped to a payment HCC. Conversely, it has added 268 new diagnosis codes that were not associated with a payment CMS-HCC in the V24 model.
4. Key Changes in V28
V28 introduced significant changes in the CMS-HCC model:
New HCCs: V28 introduced new Hierarchical Condition Categories (HCCs) that were not present in the previous versions. These new HCCs aim to capture more specific health conditions and provide a more accurate prediction of healthcare costs.
Revised HCCs: Some of the existing HCCs were revised in V28. This revision was done to ensure that the HCCs remain relevant and accurately represent the health conditions of the beneficiaries.
5. Key Differences between V28 and V24
The analysis of the CMS document highlighted several differences between V28 and V24:
HCC Count: The total number of HCCs in V28 is different from V24. This difference is due to the introduction of new HCCs and the removal or revision of some existing ones.
HCC Weights: The weights assigned to various HCCs in V28 have been adjusted compared to V24. These adjustments reflect the changing healthcare landscape and the evolving needs of the beneficiaries.
Demographic Factors: V28 has introduced changes in the way demographic factors are considered in the model. These changes aim to provide a more accurate prediction of healthcare costs based on the beneficiary's demographic details.
6. Comparison of ICD-10-CM Code Counts between V24 and V28:
The ICD-10-CM #HCC V24 model, which was in use for the year 2020, was quite extensive, encompassing a total of 9,797 codes. These codes covered a wide range of medical conditions, procedures, and other healthcare-related nuances, providing a comprehensive framework for healthcare providers and coders.
Fast forward to 2024, and the landscape appears different. The V28 model, set to be the standard for this year, has been refined and now includes 7,770 codes. This is a reduction of over 2,000 codes, representing a significant streamlining effort.
Such a substantial reduction prompts several inquiries:
Relevance and Redundancy: Were some of the codes in the V24 model found to be redundant or no longer relevant in the current medical landscape? Medical science and practices evolve, and it's possible that some conditions or procedures have been reclassified or merged, leading to the removal of certain codes.
Efficiency and Precision: The reduction might be an effort to make the coding process more efficient. By eliminating lesser-used or overly specific codes, the process can be made more straightforward without sacrificing the precision of diagnosis and treatment categorization.
Regulatory and Compliance Changes: Changes in healthcare regulations, policies, and compliance requirements can also influence the number of codes in use. The reduction might be a response to new guidelines or standards set by healthcare authorities.
Feedback and Real-world Application: The practical application of codes in real-world scenarios provides valuable feedback. Some codes might have been rarely used or found to be confusing, leading to their removal in the V28 model.
In conclusion, while the reduction in code count between the V24 and V28 models is notable, it's essential to understand that such changes are often driven by a combination of evolving medical knowledge, feedback from healthcare professionals, and the continuous pursuit of efficiency and accuracy in medical coding.
6. Implications of the Changes
The changes introduced in V28 have several implications:
Improved Accuracy: With the introduction of new HCCs and the revision of existing ones, V28 aims to provide a more accurate prediction of healthcare costs. This improved accuracy is crucial for healthcare providers and policymakers.
Better Resource Allocation: The changes in V28 ensure that resources are allocated more efficiently. By accurately predicting healthcare costs, healthcare providers can allocate resources where they are needed the most.
7. The Role of AI in Deriving Codes from Medical Records
The evolution of the CMS-HCC model underscores the importance of accurate and efficient code extraction from medical records. With the increasing complexity of medical coding and the need for precision, Artificial Intelligence (AI) offers a promising solution.
AI-Powered Medical Coding with EmedLogix NLP:
EmedLogix, leveraging the power of AI, has developed a state-of-the-art Natural Language Processing (NLP) system that can extract and interpret medical codes from vast amounts of clinical data. Here are some of its standout features:
Generative AI: EmedLogix NLP uses generative AI models that can understand the context of clinical notes, ensuring that the extracted codes are not just accurate but also relevant to the patient's condition.
Machine Learning (ML): The system continually learns from new data. As more medical records are processed, the ML algorithms become more refined, leading to even higher accuracy levels in code extraction.
Natural Language Processing (NLP): EmedLogix's NLP capabilities mean that the system can understand and interpret the nuances of clinical language. Whether it's a doctor's handwritten note or a typed discharge summary, the NLP can extract meaningful information from it.
Rules Engine: While AI and ML provide the system's intelligence, the rules engine ensures that the extracted codes adhere to the latest coding standards and guidelines. This hybrid approach ensures that the codes are not only accurate but also compliant.
In conclusion, as the healthcare industry moves towards more sophisticated risk adjustment models like CMS-HCC V28, tools like EmedLogix NLP will become indispensable. By automating the code extraction process and ensuring high accuracy levels, AI-powered systems can help healthcare providers stay compliant and ensure that patients receive the care they deserve.
8. The Future of AI in Healthcare Coding
The integration of AI into healthcare coding, as seen with tools like EmedLogix NLP, is just the beginning. Here are some potential future directions and ideas:
Predictive Analysis: Beyond just extracting codes, AI systems could predict potential future health issues based on historical data. For instance, by analyzing a patient's past medical records, AI could flag potential risks, allowing for preventive care.
Real-time Feedback: As doctors input data into electronic health records (EHRs), AI could provide real-time feedback on potential coding errors or omissions, ensuring that records are accurate from the outset.
Integration with Wearables: With the rise of wearable health devices, AI could analyze real-time data from these devices, providing immediate coding for events like heart arrhythmias or falls.
Continuous Learning from Global Data: AI systems could learn from medical records worldwide, understanding global health trends and refining coding practices based on a vast array of diverse data.
Enhanced Security: With the increasing importance of data privacy, AI can be employed to ensure that medical records are secure. Advanced algorithms can detect and prevent unauthorized access, ensuring patient confidentiality.
Automated Patient Follow-ups: Post-treatment, AI can analyze medical codes to schedule automated follow-ups with patients, ensuring they receive continuous care and adhere to medication or therapy regimens.
Training and Education: AI can also play a role in training medical professionals. By analyzing common coding errors or omissions, AI can create tailored training modules for healthcare providers, ensuring they stay updated with the latest coding practices.
In essence, the fusion of AI and healthcare coding promises not just efficiency but a revolution in patient care. As AI systems become more sophisticated, they will not only streamline administrative tasks but also play a pivotal role in patient diagnosis, treatment, and ongoing care.
9. Conclusion
The CMS-HCC model is a dynamic tool that undergoes revisions to stay relevant and accurate. The transition from V24 to V28 has brought about several changes, each aiming to improve the model's accuracy and efficiency. By understanding these changes, healthcare providers and policymakers can make informed decisions and ensure that Medicare beneficiaries receive the best possible care.
*Note: The insights presented in this article are based on the CMS document titled "Advance Notice of Methodological Changes for Calendar Year (CY) 2023 for Medicare Advantage (MA) Capitation Rates and Part C and Part D Payment Policies" and its subsequent analysis.*
Komentáře